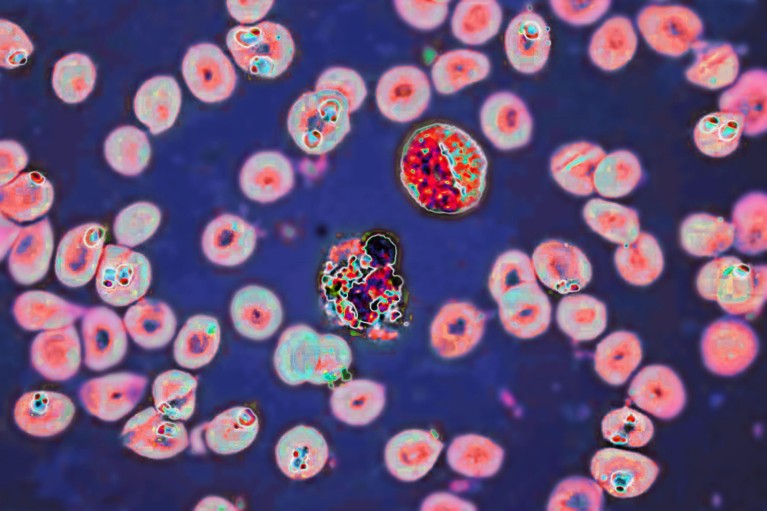
Plasmodium falciparumCredit: BSIP SA / Alamy Stock Photo
A predictive model has been developed, with potential to improve malaria diagnosis.
The model uses machine learning techniques to predict malaria incidence, taking into account clinical features, socio-demographics and environmental factors. It is not a replacement for World Health Organization (WHO)-recommended microscopy or rapid diagnostic tests, but could enhance malaria prediction and control efforts, especially given its feasibility in resource-poor settings
Olusola Ojurongbe, professor of Medical Parasitology at Nigeria’s Ladoke Akintola University ofTechnology and one of the study authors, says the benefits of accurate diagnosis are twofold: itsaves lives, especially of children with malaria, while averting unnecessary prescription of drugs andthe consequent threat of resistance.
“Malaria diagnosis in Africa is heavily reliant on Plasmodium falciparum histidine-rich protein2(PfHRP2)-based rapid diagnostic testing. However, pfhrp2 gene deletion is increasingly threateningthe sensitivity of these tests, delivering false negatives and escalating the need for complementarydiagnostic tools,” he explains.
While the findings emphasised the significance of patient characteristics including age, weight and symptoms in malaria diagnosis, along with the need for increased education of communities for malaria prediction, the study revealed associations between malaria incidence and environmental factors such as the presence of vegetation and stagnant water near or in homes.
“It was designed to augment (laboratory diagnosis) by providing an early indicator of potential disease incidence, particularly when resources for comprehensive laboratory testing are limited,” the authors, said in their paper in Biomedical Central (BMC).
Malaria is a major public health concern in Nigeria, which has one of the highest disease burdens worldwide. As many as 100 million cases are reported annually, resulting in more than 300 000 deaths.